Contents
Contents
Artificial intelligence (AI) is taking a central part of education technology, with a growing emphasis on customized learning. This approach gained tremendous traction in recent years — 97% of education leaders in a 2024 survey acknowledged the benefits of AI implementation in training programs.
EdTech companies are responding to the demand: the market for personalized learning platforms is projected to reach U.S. $72.1 billion by 2031. Such growth underscores a broad consensus that adapting content and knowledge delivery to the learner can dramatically improve engagement and outcomes.
This article explains what role AI plays in personalized learning, how it compares to traditional methods, the tech behind it, and what to consider when shaping your next steps in EdTech.
What Is Personalized Learning in Education?
Put simply, it’s a learning approach that tailors content, pace, and activities to each student’s needs. The combination of AI and personalized learning takes this further by continuously analyzing learner data and adjusting the learning journey based on their behavior, preferences, and progress.
Much like how Netflix or Spotify recommend content, AI tools for personalized learning suggest exercises and adjust instruction. EdTech platforms increasingly rely on technologies like machine learning (ML) and predictive analytics to fine-tune what content a learner sees next, whether it’s recommending additional practice on a weak skill or advancing a student to more challenging material.
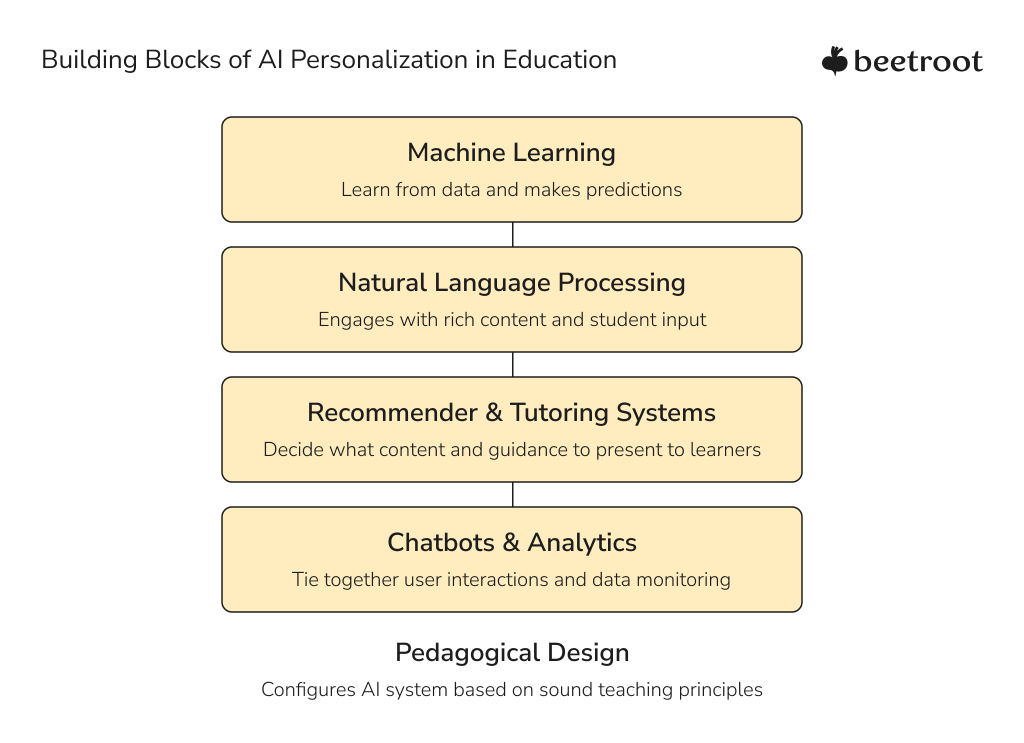
Traditional vs. Personalized Learning with AI
In traditional classrooms or static e-learning systems, all learners typically follow the same curriculum sequence and schedule, regardless of their abilities or prior knowledge. The teacher may try to differentiate instruction, but it’s challenging to do so for every student.
An adaptive LMS with AI features can automatically modify the sequence of topics, the pace of instruction, the level of difficulty, and even the type of hints or feedback provided based on each learner’s progress. If a student struggles with some prerequisite concept, AI can pause to revisit or reframe it before continuing. A traditional class would likely move on, leaving that student behind.
Aspect | Traditional Systems | AI Personalized Learning Systems |
Curriculum | Fixed sequence for all students | Dynamic, learner-specific progression |
Feedback | Periodic, generic feedback | Real-time, adaptive feedback |
Assessment | Scheduled tests | Continuous, integrated assessments |
Instructional pace | Uniform | Adaptive to each learner’s speed |
Performance Comparison
Learners often progress faster and achieve better outcomes with AI support. Research from Stanford University found that students in personalized learning environments advanced 30–40% faster through material than their peers in conventional classrooms.
Similarly, a study by the Brookings Institution found that students in schools with AI-supported assessments showed a 30% improvement in academic performance due to tailored interventions based on analytical insights.
Real-world trials echo these findings: in one experiment with working adults, an AI-powered training group improved test scores by 64%, whereas a control group with standard lectures improved by only 34%, meaning the AI-trained group outperformed the traditional approach by nearly 1.5-2x in learning gains.
While results vary by implementation, the consistent trend is that AI-driven personalization can yield significantly better learning efficiency and effectiveness than one-size-fits-all models.
Why AI Tools for Personalized Learning Matter for Business Growth
Beyond the learning benefits, AI-powered personalization carries strategic importance for EdTech companies, educational institutions, and corporate Learning & Development (L&D) programs. It can be a key driver of user engagement, customer satisfaction, and business growth.
Higher Engagement and Retention
Personalized learning experiences tend to keep learners more engaged in both academic and corporate settings. Companies with tailored learning programs display notable boosts in employee engagement – a 17% increase, according to Deloitte.
Predictive analytics can flag at-risk students or employees early, allowing timely support. Adaptive LMSs with AI tools for personalized learning and assessment have seen a 40% improvement in student retention rates, indicating far fewer students giving up on courses.
Engagement has direct business implications: Docebo reported that 58% of employees are more willing to engage with training content that is personalized and delivered in shorter, digestible units. Engaged and successful learners are more likely to continue using the platform, pursue additional courses, or advocate for it, expanding your product’s reach.

Competitive Advantage and Market Demand
AI and personalized learning solutions are becoming a must-have in the increasingly crowded EdTech market, and platforms offering advanced personalized learning programs are gaining a distinct advantage.
Analysts forecast the global market for personalized learning with AI products to reach U.S. $72 billion by 2031. Organizations that fail to integrate AI agents in EdTech or modernize their products with AI capabilities risk falling behind as user expectations shift toward more tailored, flexible experiences.
In short, personalized learning with AI is becoming a baseline expectation for learning experiences across sectors.
Better Learning Outcomes and ROI
Adopting AI personalized learning systems results in tangibly improved learning outcomes, which directly translate to better business metrics. Personalized programs often lead to:
- Faster competency development (up to 30–60% acceleration in skill acquisition)
- Higher course completion rates
- Improved learner satisfaction scores
Personalized training means people learn what they need in less time. For corporations, this means faster onboarding, higher productivity, and reduced training costs. For EdTech providers, better learner outcomes mean stronger case studies, higher platform stickiness, and improved customer lifetime value.
The benefits of personalized learning programs powered by AI are clear: more engaged users, better skill mastery, and a demonstrably higher return on investment.
Key Technologies Behind Personalized Learning Platforms
Delivering personalized learning at scale is a technically complex task made possible by a convergence of advanced AI technologies. Core components include:
Machine Learning Algorithms
Machine learning (ML) is the engine of personalization. ML models analyze student data (quiz results, click patterns, time spent, etc.) to detect learning patterns and predict needs. ML-based analytics can forecast which topics a learner might struggle with and proactively adjust the curriculum, such as serving easier or harder questions depending on their performance, to address knowledge gaps and challenge them appropriately.
Natural Language Processing (NLP)
In personalized learning, NLP powers intelligent tutoring systems that can converse with a student in natural language. NLP also helps analyze textual resources: it can summarize or simplify complex reading materials or even translate content into a student’s native language.
Modern AI chatbots (like those built on GPT-4 or similar large language models) are an extension of NLP, capable of providing on-demand hints, feedback on essays, or answers to questions in a conversational manner.

Recommender Systems
Recommender systems in EdTech function much like those in e-commerce or media. They analyze user history, content engagement, and performance data to suggest the next best learning activities. If a student is preparing for a certification, the system might recommend specific modules they haven’t mastered yet or suggest more video content if data shows they learn better via videos than text.
In corporate environments, recommenders help employees find precisely the microlearning they need to address specific skill gaps, supporting the broader strategy of personalized learning programs.
Intelligent Tutoring Systems (ITS)
An ITS is an AI-driven learning coach that provides one-on-one instruction and feedback. These systems combine domain expertise (often a model of the subject matter), student modeling, and tutoring strategies. They continuously adapt to a learner’s input, offering relevant hints, solutions, or new problems and building a profile of the student’s knowledge state over time.
An example like Carnegie Learning’s MATHia shows how an ITS can replicate aspects of human tutoring at scale, which is why they’re considered a pinnacle of AI personalization in learning.
Chatbots and Virtual Assistants
These are AI agents that support learners (and sometimes instructors) by automating interactions. In an educational context, chatbots can handle FAQs, quiz a student with flashcards, or even simulate a dialogue for language practice.
Unlike human instructors, chatbots are available 24/7, giving students help at any hour. While not as advanced as full ITSs, virtual assistants add a layer of personalized support and remove minor bottlenecks like waiting for a teacher’s email response, thereby keeping learners on track.
Data Analytics and Learner Modeling
Underlying all of the above is a robust data infrastructure. AI personalization relies on aggregating and analyzing large amounts of learner data — every click, response, and interaction can be informative. Platforms often maintain a learner model for each user, which is essentially a data-driven profile of their knowledge, skills, and preferences.
Techniques such as:
- Bayesian knowledge tracing,
- Knowledge graphs mapping concept dependencies,
- Adaptive learning algorithms,
ensure the AI’s decisions (what to recommend next, whether to review a topic, etc.) are grounded in evidence. The result is a continuously updating personalized learning plan for each student, recalculated as new data comes in.
Building Personalized Learning Paths with AI
One of the practical aims of AI in education is to construct personalized learning paths – the sequence of learning activities uniquely suited to each learner’s progress. Here’s how AI and personalized learning typically work together to build them:
1. Mapping the Learning Domain
Educators or content developers define the learning objectives, often creating a knowledge graph or curriculum chart that shows how concepts are interrelated before AI can personalize a journey. This map serves as the playground for the AI – it knows the possible routes a student can take through the material and the dependency of topics.
Personalized learning platforms such as Khan Academy use structured skill maps to guide learners logically through mathematics skills, ensuring that the learning progression respects prerequisite relationships.
2. Assessing the Learner and Collecting Data
Once the structure is in place, the next step is to measure the learner’s prior knowledge. Diagnostic tests, adaptive assessments, or first interactions help shape the initial profile. As the learner engages with the system, AI continuously collects performance data (quiz scores, time taken per question, content items accessed, even keystroke dynamics) and feeds them into this learner model, developing a detailed profile of the student’s strengths and weaknesses over time.
3. Identifying Gaps and Adapting the Path
Using the data, the AI detects what each learner needs to work on. Techniques like Bayesian knowledge tracing come into play here: the system statistically estimates the probability that the student has mastered each knowledge component, updating these estimates every time the student answers a related question.
This is where AI’s ability to personalize becomes very tangible. Instead of a fixed syllabus, the learner’s path adjusts based on performance, whether it’s revisiting earlier units, spending more time on fundamentals, or skipping mastered topics. The platform builds a custom lesson plan for each session, drawn from the broader curriculum but focused on unresolved skills.
4. Continuous Feedback and Iteration
Immediate, granular feedback is a hallmark of AI-powered systems. This real-time feedback loop keeps students informed of their progress and can motivate them by showing incremental achievements (like earning a mastery badge for a topic).
As the learner progresses, the AI updates the learner model continuously, adjusting content recommendations. For instance, if a student quickly masters a skill flagged earlier as difficult, the AI can fast-track their progression to more complex material.
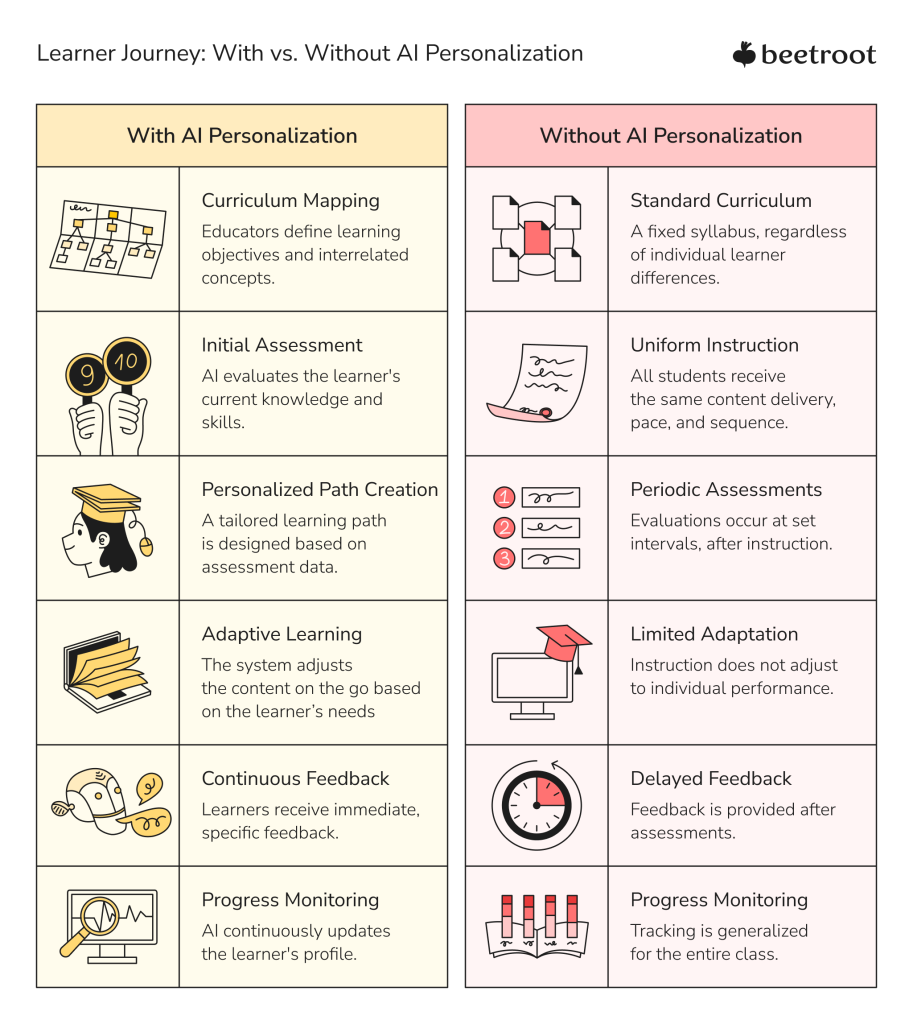
Example – A Personalized Path in Action
To illustrate, consider Duolingo, a popular language-learning app. Duolingo’s AI system collects hundreds of millions of data points from its 41 million daily active users. It adapts each learner’s schedule individually, recommending practice at exactly the optimal time to prevent memory decay. This adaptive approach, grounded in spaced repetition principles, is designed to enhance long-term vocabulary retention compared to fixed review schedules.
Workplace L&D programs leveraging AI-driven adaptive learning have reported significant improvements in learner engagement and training effectiveness as well. For instance, a LinkedIn Learning study noted a 45% increase in engagement among employees following personalized learning paths. On top of that, companies have seen up to a 30% boost in training effectiveness and knowledge retention when implementing AI-personalized learning strategies.
Common Implementation Challenges
Despite AI’s promises, implementing smart solutions comes with a set of hurdles that slow broader adoption of personalized learning systems. Here’s what you’ll want to keep in mind as you plan, build, or scale your AI-driven learning feature:
Data Privacy and Security
Personalized learning systems process large amounts of learner data, from quiz scores to behavioral data. Depending on your region, regulations like FERPA (in the U.S.) or GDPR (in the EU) apply. In K–12 settings, additional consent from parents may be required. Make sure you’re using strong encryption, secure storage, and clear, transparent policies. Responsible use of data is both a technical and ethical obligation.
Algorithmic Bias and Fairness
Even with good intentions, AI can replicate bias if it’s trained on incomplete or skewed data. If your system is recommending easier content more often to certain learners or misinterpreting second-language speakers’ responses, it could unintentionally reinforce inequality. To avoid this, models must be trained on diverse datasets and regularly audited. It also helps to provide users with clear explanations for AI-generated decisions.
Teacher Training and Buy-In
No AI system will succeed in a learning environment without educator support. If teachers or trainers aren’t involved in implementation or don’t trust the tool, you’ll likely face resistance. Early buy-in is key. Give educators access to real results, offer training that focuses on how AI complements (not replaces) their work, and involve them in shaping how the system is used.
Technical Integration and Scalability
From a technical standpoint, scalability and integration can make or break your implementation. AI models, especially those that deliver real-time feedback or personalized content, require solid infrastructure. Cloud-based solutions can help, but they still need to integrate smoothly with your existing LMS or tools. Without that integration, you risk creating data silos and extra work for your team. Also, AI performs best with well-structured and tagged content. If manual reformatting is needed, plan for it in advance and clarify long-term maintenance costs.
Ethical and Pedagogical Concerns
AI should support, not replace, human teaching. While it can automate tasks and personalize learning, it lacks the judgment and empathy of educators. Students should retain some choice and understand how and why the AI is making recommendations, and educators should still have the ability to override the system when needed. Ethical implementation means giving users transparency and autonomy.
Make it clear when AI is making a decision and why. More institutions are moving toward a “human-in-the-loop” approach, where the AI handles automation, but educators remain in control. It’s a smart balance that protects learning quality and keeps your platform aligned with emerging standards.

Case Studies: Personalization in Action
Real-world applications of AI personalization show how adaptive systems can improve learning outcomes, increase efficiency, and scale across different contexts. Below are key highlights from both education and corporate training.
K–12: Mastery-Based Learning with Khan Academy
Khan Academy’s AI-powered Mastery Learning platform helps students progress at their own pace by identifying and addressing individual skill gaps. In a study across 47 U.S. schools using the MAP Accelerator, students who engaged with the platform for 30 minutes or more per week achieved 20% higher-than-expected gains in math performance compared to non-users. Teachers also reported spending less time on basic instruction, freeing them to focus on individual support.
Higher Education: Adaptive Courseware at Arizona State University
To improve outcomes in high-enrollment, high-failure courses, ASU integrated adaptive learning into subjects like College Algebra, Microeconomics, and Biology. After the shift:
- Success rates in College Algebra rose from 57% to 85%.
- In Microeconomics, A grades tripled, while failing grades dropped sharply.
- In Biology, students earning a C or better increased from 72% to 90%.
These results highlight how adaptive courseware, combined with thoughtful instructional design, can significantly boost academic performance at scale.
Secondary Education: AI Tutoring with MATHia by Carnegie Learning
Carnegie Learning’s MATHia platform offers AI-powered, step-by-step math tutoring that adjusts in real time based on each student’s progress. It’s designed to mirror the feedback and pacing of a human tutor while giving educators detailed dashboards to monitor learning.
In a recent statewide review, each 10-point increase in MATHia’s APLSE score (a measure of how well a student is progressing) correlated with a 0.13 to 0.25 standard deviation increase in end-of-year test scores.
Corporate L&D: AI-Powered Training with Docebo LMS
A global technology company adopted Docebo’s AI-driven learning management system to personalize training based on job roles and learner behavior. After implementation, the company saw a 45% boost in training engagement and a 30% decrease in average course completion time. The system also improved the learner experience by recommending content at the right time and skipping material already mastered.
Professional Development: Adaptive Learning with STAI
Smart Training AI (STAI), a London-based EdTech company, conducted a landmark study with a professional development course in the Middle East to quantify the impact of AI personalization. In a controlled study with 257 professionals, STAI compared its AI-powered adaptive platform to traditional training. The AI group saw a 64% improvement in test scores versus 34% in the traditional group — an 88% higher performance gain. The platform tailored content to each learner’s needs and preferences, leading to better engagement and faster skill development.
Workplace Training: AI-Driven Onboarding and Performance Gains
Insights from Unboxed Training & Technology, Deloitte, and Harvard Business Review show that AI-personalized training is improving both the speed and quality of workforce development. A Deloitte case found that using an AI-based recommendation system reduced time-to-competency by 20% for new hires by tailoring training to each employee’s skill level and pace. Meanwhile, HBR reports link AI-enhanced learning to a 25% improvement in process efficiency and a 22% boost in employee satisfaction as learners felt better supported through more relevant, timely content.
Across these case studies, a few common themes emerge:
- Context matters: the best results come when AI personalization is integrated thoughtfully into the curriculum or training program (often as part of a blended model with human facilitation).
- Success is typically measured in improved learning outcomes (test scores, proficiency gains, completion rates) and/or efficiency gains (reduced time, higher engagement) – and the above examples show significant improvements in both dimensions.
Lastly, they illustrate that AI personalization can be effective in diverse settings: young students learning basic skills, college students in large classes, or professionals upskilling on the job.
Conclusion
AI-driven personalization is changing the way people learn — helping individuals progress faster, close knowledge gaps, and stay engaged. As the examples above show, when thoughtfully applied, these systems can make a meaningful impact in both education and workplace learning.
Adopting AI in learning is about more than choosing the right tools. It’s also about how technology fits into real teaching and training contexts. At Beetroot, we combine hands-on software development experience with a strong foundation in education. Through Beetroot Academy, we help teams build practical skills through expert-led training — whether for product development, platform integration, or team upskilling.
If that’s something you’re exploring, feel free to get in touch with our experts to see how we can help.
Subscribe to blog updates
Get the best new articles in your inbox. Get the lastest content first.
Recent articles from our magazine
Contact Us
Find out how we can help extend your tech team for sustainable growth.