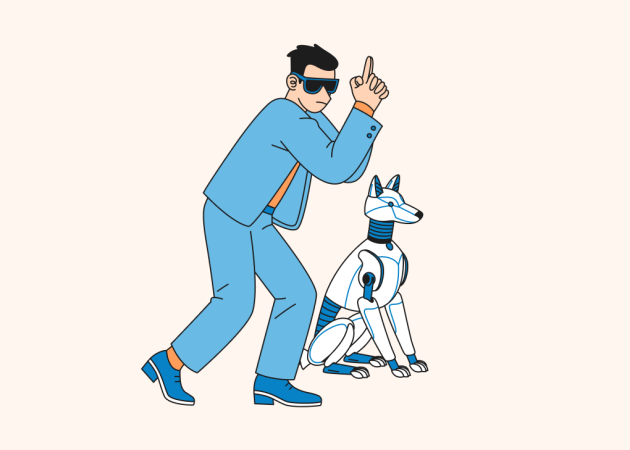
AI and Cybersecurity: Roles, Threats, Solutions, and Future
Contents
In today’s digital world, AI and cybersecurity have become tightly connected, bringing both exciting possibilities and complex challenges for businesses. On one hand, AI offers powerful tools to detect, analyze, and counter cyber threats faster than ever before. On the other hand, it also brings new risks. For example, generative AI can be used by bad actors to create advanced phishing schemes, malware, and even deepfakes.
Businesses need strong cybersecurity solutions to tackle today’s risks and threats. Despite the obstacles, the potential for AI in cybersecurity is enormous. According to Statista’s 2024 overview, the market is expected to grow from $24 billion in 2023 to an estimated $134 billion by 2030. The risks of cybersecurity will shape not only the future of this field but also influence broader business strategies that rely on digital trust.
In this article, we dive into the dual role of AI in cybersecurity, examining both the opportunities and the challenges ahead.
The Role of AI in Cybersecurity
Artificial Intelligence (AI) is now a key player in cybersecurity, bringing advanced tools to identify, analyze, and respond to cyber threats. AI’s role in artificial intelligence and security extends beyond reactive measures and encompasses predictive capabilities that help organizations anticipate and prevent attacks. Here are some ways AI enhances cybersecurity:
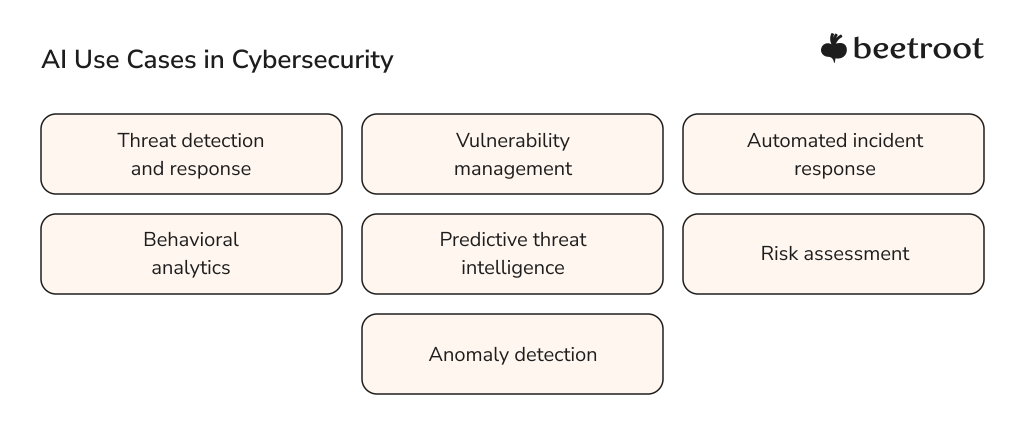
- Threat detection and response: By processing large amounts of data, AI identifies patterns that suggest cyber threats. Analyzing network traffic and user behavior, it spots unusual activity that could signal attacks, allowing for quick action to mitigate potential damage.
- Vulnerability management: AI continuously scans for weaknesses and helps prioritize them based on potential impact. This proactive monitoring enables organizations to address critical issues before they can be exploited.
- Automated incident response: AI-driven tools can automate responses to certain incidents, minimizing damage and reducing the time between detection and remediation. This quick response helps restore normal operations promptly.
- Behavioral analytics: AI establishes baselines of normal user behavior, allowing it to detect deviations that might indicate compromised accounts or insider threats, enhancing security against unauthorized access.
- Predictive threat intelligence: By analyzing both historical and real-time data, AI identifies trends and patterns to forecast potential cyber threats. This predictive capability empowers organizations to reinforce defenses against future attacks.
- Risk assessment: AI evaluates the likelihood and potential impact of various threats, guiding organizations in prioritizing security measures and resource allocation. This strategic approach bolsters overall security resilience.
- Anomaly detection: AI continuously monitors for unusual system behavior, detecting early signs of emerging threats. This enables timely intervention to prevent breaches before they escalate.
Incorporating AI in cybersecurity examples like these can help organizations strengthen their defenses, stay ahead of potential threats, and address risks before they arise. This dual role — being both reactive and predictive — highlights AI’s transformative impact on the cybersecurity landscape.

Key Threats in AI-Driven Cybersecurity
In the evolving landscape of cybersecurity, AI cybersecurity threats are quickly becoming a daily reality. Most security leaders expect to face AI-based attacks regularly, with two-thirds believing that AI will soon be a staple in the cybercriminal toolkit. This “offensive AI” is seen as a game-changer, enabling faster, more sophisticated attacks that are increasingly difficult to counter.
Despite the surge in offensive AI, there remains some confusion over which threats pose the most significant risks. Bot attacks, for example, are often underestimated — they can cost large businesses up to 4.3% of their online revenue. Interestingly, while AI for threat detection is enhancing security by decreasing operational costs and improving defensive measures, it’s mostly being applied to high-impact, low-frequency attacks like DDoS, rather than the more frequent bot attacks. This gap suggests that while AI is bolstering defenses, it’s not yet optimized across the board.
Here are some of the most pressing AI cybersecurity threats:
- AI-Powered Cyberattacks: Just as AI strengthens defenses, it can also supercharge cyberattacks. Attackers are increasingly using AI to automate tasks that traditionally required significant manual effort, such as scanning for vulnerabilities, crafting phishing emails, or identifying exploitable weaknesses within systems. This automation speeds up attacks and allows them to occur on a much larger scale. With AI, attackers can develop more sophisticated and targeted approaches, making it more challenging for organizations to keep up with evolving threats.
- Adversarial AI: Adversarial AI is a tactic where attackers exploit AI’s own algorithms by feeding it carefully manipulated data that causes it to make incorrect decisions. For instance, adversarial examples could trick a malware detection system into identifying malicious files as harmless. These subtle alterations can bypass defenses without raising red flags. In cybersecurity, adversarial attacks are particularly dangerous because they undermine the very tools meant to protect systems, and they are difficult to detect without specialized countermeasures.
- Deepfake Technology: Deepfake technology is another emerging threat enabled by AI. Using machine learning, attackers can generate hyper-realistic synthetic media, like videos and voice recordings, that convincingly impersonate real individuals. Cybercriminals use deepfakes for social engineering schemes, such as impersonating a CEO to authorize a fraudulent transaction or creating fake identities to gain access to restricted information. The high realism of deepfakes makes them a potent tool for deception, posing significant risks to organizations.
- Ethical AI Vulnerabilities: Ethical vulnerabilities in AI, such as biases in data or “black-box” decision-making, pose another layer of risk. AI algorithms rely on large datasets, and any biases in these datasets can result in errors, such as incorrectly flagging safe activity as malicious or missing true threats. Additionally, AI systems can be opaque in their decision-making processes, making it hard for security teams to understand why an AI has made a particular choice. This lack of transparency can slow responses to threats and decrease trust in AI-driven cybersecurity solutions. Privacy concerns also arise, as AI often needs access to large volumes of data, increasing the risk of sensitive information exposure if these datasets are compromised.
Examples of AI-Powered Solutions for Cybersecurity
From spotting vulnerabilities to detecting phishing schemes, AI provides tools and insights that would be nearly impossible to achieve manually. Here’s how AI is revolutionizing cybersecurity in several key areas:
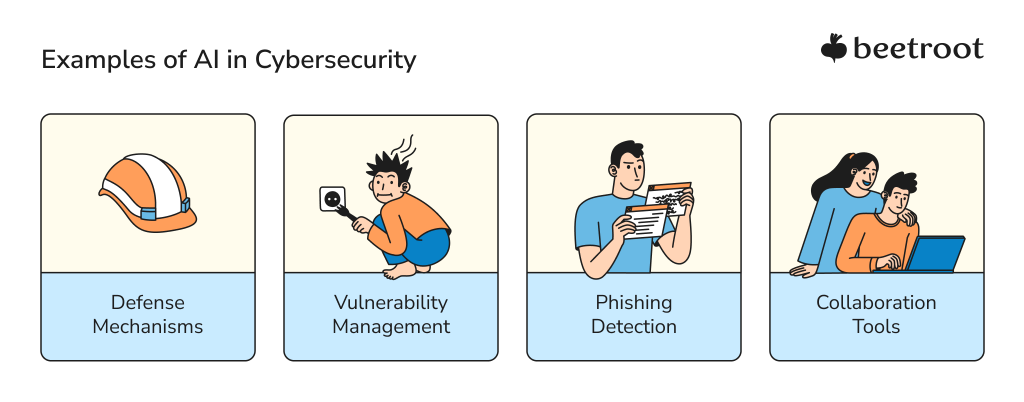
AI-Based Defense Mechanisms
One of the primary roles of AI for defensive cybersecurity is fortifying defense mechanisms. AI-based tools enhance detection and response times by continuously monitoring networks and identifying unusual activities. This approach, known as behavioral analysis, allows AI to establish a baseline of normal operations within a system, making it easier to detect anomalies that could indicate a cyber threat. When these systems detect suspicious behavior, they can automatically trigger alerts or even initiate response protocols to contain potential threats in real-time.
Machine learning algorithms also strengthen AI-based defense mechanisms by adapting over time. As these algorithms process more data, they become better at recognizing and responding to new types of attacks. The ability to learn from past incidents means that AI systems can quickly evolve to stay ahead of attackers, making them highly valuable in maintaining a robust cybersecurity posture.
AI for Vulnerability Management
The use of AI in cybersecurity has been a game-changer for vulnerability management, helping organizations proactively identify and prioritize weaknesses within their systems. Vulnerability management requires continuous scanning and analysis, which is both time-consuming and prone to human error. AI for vulnerability management simplifies this process by automating scans and assessing vulnerabilities based on their risk level.
For example, AI algorithms can rank vulnerabilities according to factors like the exploitability of the flaw, the level of access an attacker would need, and the potential impact on the organization. This prioritization helps security teams focus on the most critical vulnerabilities first, ensuring that high-risk issues are addressed promptly. Additionally, AI can automate patch management, enabling faster application of security updates to systems and reducing the risk of attack on known vulnerabilities.
AI for Phishing Detection
Phishing is one of the most common and damaging cyberattack methods, often targeting employees through deceptive emails or messages. AI is proving to be a powerful tool for spotting phishing attempts before they reach their targets. AI for phishing detection works by analyzing vast amounts of data, looking for telltale signs of phishing, such as unusual language, email patterns, or sender anomalies.
Machine learning models can be trained on known phishing examples, allowing them to distinguish between legitimate and suspicious communications. Some advanced AI-powered email filters go beyond scanning for known threats by analyzing the behavior of the sender and the email content, identifying potential phishing attempts that might slip through traditional filters. This proactive approach significantly reduces the risk of successful phishing attacks, protecting users and sensitive information from falling into the hands of cybercriminals.

AI-Driven Collaboration Tools
AI cybersecurity solutions are transforming collaboration tools by integrating data sources and providing security teams with actionable insights. Tools like IBM QRadar Advisor with Watson and Microsoft Azure Sentinel bring together threat intelligence, data analytics, and machine learning to streamline cybersecurity efforts.
- IBM QRadar Advisor with Watson combines IBM’s SIEM system with Watson’s AI capabilities to analyze and contextualize threats. It automatically investigates incidents, providing teams with insights into potential attack methods. By processing vast amounts of data, it identifies correlations and delivers actionable recommendations, reducing response times.
- Microsoft Azure Sentinel is a cloud-native SIEM and SOAR tool that uses AI to detect and respond to threats across an organization’s infrastructure. Leveraging Microsoft’s data resources and machine learning, it provides real-time insights and integrates seamlessly with third-party tools, automating common security tasks to help teams manage threats efficiently.
These AI-driven collaboration tools not only make it easier for security teams to identify and respond to threats but also foster a proactive approach to cybersecurity, where threats are anticipated and addressed before they can escalate.
By enhancing defense mechanisms, simplifying vulnerability management, detecting phishing attempts, and streamlining collaboration, AI is helping businesses build a more resilient security posture in the face of evolving cyber threats. As cybercriminals continue to adopt AI for their own attacks, AI-powered defense strategies are essential for staying one step ahead.
Challenges of Implementing AI in Cybersecurity
While AI in cybersecurity offers major benefits, implementing it effectively presents a unique set of challenges, from high costs to ethical concerns. Here’s a concise look at the hurdles organizations face with AI-driven cybersecurity and how they can address them.
High Initial Costs and Resource Demand
Implementing AI cybersecurity solutions requires substantial investment in both technology and skilled personnel. Custom setups or configurations to meet specific needs can further raise costs. For smaller businesses, these costs pose a particular challenge, making sustainable AI for cybersecurity essential. Cloud-based solutions like Microsoft Azure Sentinel and IBM QRadar offer a scalable, pay-as-you-go model, making AI more financially accessible and sustainable.
Data Privacy and Security Risks
AI-driven systems need large volumes of data for effective threat detection, which presents privacy risks if these datasets are compromised. Sensitive data could become a target, adding a new layer of vulnerability. Organizations must employ strong data protection strategies, such as encryption, anonymization, and strict access controls, and perform regular audits to ensure privacy standards remain high.
Ethical AI in Cybersecurity
Ethical AI addresses fairness, transparency, and bias in AI models, crucial in cybersecurity decision-making. Models trained on biased data can lead to unfair or ineffective outcomes, such as missing critical threats or flagging benign activity as suspicious. Using explainable AI (XAI) and regularly evaluating AI systems for bias can help maintain fair, ethical standards, ensuring that AI operates transparently and reliably.
Complexity in Integration and Compatibility
Integrating AI into diverse tech environments can be challenging, especially with legacy systems. Effective deployment often requires AI to access multiple data sources and communicate across systems. To ease integration, organizations should select AI solutions with strong interoperability, using flexible API integrations to connect with existing tools.
Skills Gap and Training Needs
Many organizations lack the specialized expertise needed for AI-driven cybersecurity, exacerbating resource demands on already stretched security teams. Investing in training programs to upskill current employees or partnering with third-party AI specialists can help bridge this skills gap and build in-house capabilities over time.

Sustainability and Environmental Impact
AI’s computational demands can lead to high energy consumption, making sustainable AI for cybersecurity a priority. Environmentally conscious organizations are exploring ways to balance AI’s benefits with their sustainability goals.
Adopting energy-efficient AI solutions, choosing cloud providers with renewable energy commitments, and optimizing AI algorithms for reduced computational demands can help achieve more sustainable AI use.
False Positives and Alert Fatigue
AI-based systems can produce false positives, overwhelming security teams with unnecessary alerts and potentially causing them to overlook actual threats. Organizations can combat alert fatigue by prioritizing alerts based on severity and likelihood, fine-tuning AI models, and adjusting sensitivity to reduce false positives.
Ongoing Maintenance and Model Updates
AI models require continuous updating to stay effective against evolving threats. Regular maintenance, retraining, and tuning are necessary but resource-intensive. Automating model updates and partnering with vendors offering ongoing support can ease this burden, ensuring AI systems remain current and effective with minimal manual intervention.
The Future of AI in Cybersecurity – Key Trends and Forecasts
AI in cybersecurity is transforming how businesses detect, prevent, and respond to threats. Its ability to analyze vast amounts of data, recognize patterns, and adapt to new attack methods makes it indispensable in today’s evolving threat landscape. Here’s a look at key trends shaping the future of AI in data security:
- Predictive threat detection: AI’s predictive capabilities allow organizations to anticipate cyber threats before they occur. By analyzing historical and real-time data, AI can identify trends and recognize attack patterns, helping businesses stay one step ahead of cybercriminals.
- Increased use of AI-driven automation: Automation is streamlining threat detection, incident response, and vulnerability management, reducing the need for manual intervention. This is especially beneficial as cyber threats become more complex, allowing security teams to focus on high-priority issues.
- Rise of ethical and explainable AI: As AI takes on a larger role, businesses are prioritizing transparency and fairness in AI models. Explainable AI (XAI) is becoming essential for understanding AI-driven decisions, building trust, and ensuring ethical practices in cybersecurity.
- Scalable AI for small and medium businesses: AI solutions are increasingly accessible, with cloud-based models allowing businesses of all sizes to leverage advanced cybersecurity measures without heavy upfront investments. This trend will expand as more scalable, budget-friendly AI tools become available.
As AI continues to evolve, its role in cybersecurity will grow, making it an essential component for safeguarding digital assets and business operations.
Beetroot is a trusted tech partner for businesses seeking robust cybersecurity and AI expertise. With deep technical skills and a focus on sustainable, ethical solutions, Beetroot supports companies in building scalable cyber security frameworks through team extensions, project-based software development, and cybersecurity workshops for teams. Whether you’re looking to enhance your cybersecurity or explore AI-driven innovations, Beetroot’s team is ready to help you stay secure and competitive.
FAQ
How can AI improve threat detection in cybersecurity?
AI boosts threat detection by spotting unusual patterns in massive amounts of data, flagging potential risks faster and more accurately than humans. It helps identify threats before they can cause damage, giving security teams a major advantage.
How can AI help prevent future cyberattacks?
AI can predict and prevent future attacks by analyzing past incidents and real-time data. It learns from previous threats to recognize new ones, helping organizations strengthen their defenses and stay a step ahead of attackers.
Is AI in cybersecurity sustainable for small businesses?
Yes, many AI-powered cybersecurity tools are designed to be accessible and affordable for small businesses. Cloud-based options and scalable solutions make it easier for smaller teams to implement AI-driven protection without breaking the bank.
What is adversarial AI, and how does it threaten cybersecurity?
Adversarial AI involves attackers feeding misleading data to AI systems to trick them. This can cause security tools to overlook threats or flag safe files as dangerous, making it a serious risk for cybersecurity systems.
Subscribe to blog updates
Get the best new articles in your inbox. Get the lastest content first.
Recent articles from our magazine
Contact Us
Find out how we can help extend your tech team for sustainable growth.