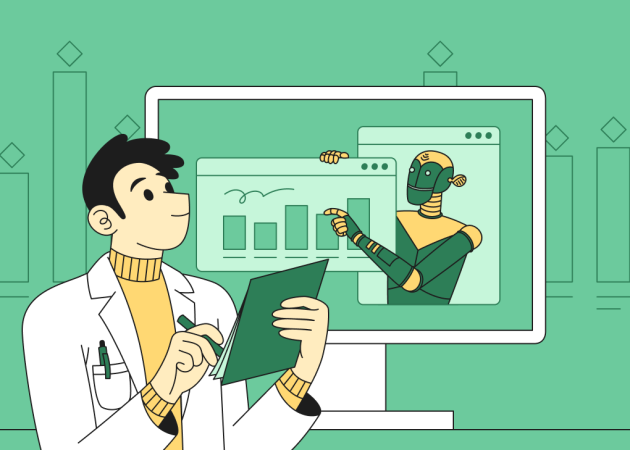
From Data to Decisions: The Role of AI Predictive Analytics in Healthcare
Contents
Contents
TL;DR
AI predictive analytics in healthcare involves using machine learning (ML) algorithms and statistical algorithms to analyze historical and real-time healthcare data. It predicts future outcomes such as disease progression, hospital readmissions, or patient deterioration, enabling proactive interventions. By shifting from reactive to preventive care, predictive analytics supports better decision-making, optimized operations, and improved patient outcomes.
Introduction
Predictive modeling is increasingly used in healthcare to convert large datasets into actionable insights. By analyzing electronic health records (EHR), clinical results, and patient information, healthcare providers can forecast patient outcomes, shifting from reactive to proactive care. Approximately 65% of U.S. hospitals already integrate predictive models into their EHR systems.
What is AI Predictive Analytics in Healthcare
AI predictive analytics employs advanced data analysis techniques, including statistical algorithms and machine learning, on current and historical healthcare data to predict future events or outcomes. In practice, this means mining datasets (e.g., patient records, claims, imaging, lab results) to identify patterns and forecast things like disease progression, hospital readmission risk, or treatment responses.
Predictive analytics supports initiatives in healthcare software development that aim to build smarter healthcare ecosystems. Unlike traditional decision-making reliant solely on clinician expertise, AI predictive analytics uses evidence-based, real-time insights for proactive patient care.
Benefits of Predictive Analytics in Healthcare Ecosystems
AI predictive analytics offers numerous benefits to care delivery organizations as well as the technology companies that serve them:
Improved Patient Outcomes
A core advantage is the ability to detect patient risks early and intervene sooner. Predictive models can flag high-risk patients before symptoms escalate, enabling timely treatment that can avert complications.
For example, NYU researchers developed an AI model that accurately predicts 80% of patient readmissions by analyzing unstructured EHR notes, outperforming existing methods by 5%.
In another case, Corewell Health (Michigan) applied predictive analytics to identify recently discharged patients at high risk of readmission, preventing 200 hospital readmissions over 20 months and saving an estimated $5 million in costs.
Enhanced Clinical Decision Support
Predictive analytics provides an added layer of decision support for clinicians. AI tools for early disease detection help uncover subtle indicators a human might miss. By crunching complex datasets (e.g., vital signs, lab trends, medical history), risk-scoring models can stratify patients by risk level.
These risk scores help care teams personalize treatment plans, paying extra attention to patients flagged as high risk. It’s especially useful in managing chronic diseases like diabetes: predictive algorithms can forecast which patients are likely to experience issues like kidney problems or hospitalizations, prompting preventive measures far in advance.
Operational Efficiency
Predictive analytics helps hospitals and clinics optimize everything from staffing to supply chain by forecasting future needs. One example is AI-driven scheduling tools that analyze historical patient volumes, seasonal trends, and real-time data to allocate the right number of staff at the right times.
By predicting admission rates and length of stay, hospitals can optimize bed management and reduce bottlenecks in emergency departments. AI systems can also forecast the usage of medications and supplies, reducing inefficiencies. Industry reports from the U.S. indicate that smart data strategies can save the healthcare system up to $100 billion annually.
Population Health Management
AI-driven analytics allows healthcare providers to identify population-level trends and high-risk cohorts. This is valuable for value-based care initiatives focused on keeping patient populations healthier and out of the hospital.
Predictive analytics supports programs in chronic disease management and preventive screenings by zeroing in on who would benefit most. These efforts improve outcomes at a population level and can reduce disparities in care provision.
Benefits for HealthTech Solution Providers
The importance of predictive analytics in healthcare is growing. Hospitals and clinics increasingly expect their EHR systems and digital health tools to include predictive capabilities, and 79% of those already using predictive analytics built their models with their EHR development vendor.
This means EHR and HealthTech providers who offer AI-driven analytics can capitalize on a fast-growing demand by delivering immediate value to clinical users. The market for healthcare predictive analytics is forecast to grow more than tenfold from ca. $16.8 billion in 2024 as more providers invest in the technology.

How AI is Transforming Predictive Modeling in Healthcare
AI is enhancing traditional predictive models by incorporating broader data sets, including genomics and social determinants of health. One of Beetroot’s long-term partners is a renowned provider of evidence-backed XAI (explainable AI) solutions for genomic diagnostics and discovery — read more about our cooperation here.
Predictive tools in healthcare now rely on large-scale data integration, real-time health monitoring, and advanced learning algorithms. Solutions like AI in mental health care are early examples of predictive models improving outcomes by detecting mental health risks sooner.
AI health monitoring systems, such as wearable-enabled platforms, provide continuous data streams that feed predictive algorithms, allowing real-time alerts and interventions.
Predictive Analytics in Healthcare: Examples & Use Cases
Healthcare teams apply AI predictive analytics across a wide range of clinical and operational use cases. Leading medical AI startups continue to drive advances, shaping the future of healthcare. Some prominent examples include:
Patient Risk Stratification and Early Intervention
One of the most common applications is predicting patient risks and health trajectories for inpatients. A notable example is Kaiser Permanente’s deployment of an early warning AI system for inpatient deterioration. It analyzes vital signs and lab results in real time and alerts a rapid response team if a patient is likely to decline. In a multi-hospital study, this approach was associated with a 16% reduction in hospital mortality among the intervention group.
Beyond the hospital walls, analytics can also identify high-risk outpatients: 79% of hospitals in one study used models to pinpoint which recently discharged or chronic patients were most likely to need follow-up care or readmission. By knowing who is at greatest risk (for example, a chronic heart failure patient at risk of an ER visit), clinics can target those individuals with extra care coordination, check-ins, or preventive treatments to avoid acute incidents.
Chronic Disease Management and Readmissions Prevention
Algorithms can predict how likely a patient with a chronic condition (e.g., diabetes, COPD, or heart failure) can experience a severe exacerbation in the near future. Providers use these predictions to tailor interventions (e.g., scheduling a sooner follow-up, adjusting medications, or arranging home health support) to prevent the predicted outcome.
A practical use case is hospital readmission prediction: many health systems now deploy machine learning models that estimate a patient’s 30-day readmission risk at discharge. If the risk is high, case managers will intervene with enhanced discharge planning and post-discharge support (home visits, phone check-ins, etc.).
Predictive analytics is also strengthening chronic disease management. By tracking data from wearables, remote monitors, and health records, it can alert providers when a patient shows early signs of trouble like worsening heart failure seen in weight or blood pressure changes. This gives clinics a chance to adjust treatment or step in early, often preventing hospitalization.

Resource and Operations Optimization
Beyond patient care, hospitals use AI predictive analytics to run operations more smoothly. Staffing and scheduling are major areas: predictive models learn from historical data, seasonal trends, and local events to forecast needs, helping avoid understaffing, overtime, or last-minute scrambles.
For example, AI scheduling tools can predict a surge in ER visits over a holiday weekend and recommend adjusting staff in advance. Hospitals also use predictive analytics to manage patient flow — forecasting admissions, discharges, and ED arrivals to allocate beds and resources proactively. Operating rooms benefit, too, with models predicting case durations and scheduling add-ons more efficiently.
On the supply side, algorithms forecast when critical inventory will run low, allowing hospitals to restock before shortages happen. During the COVID-19 pandemic, predictive models helped many health systems manage ventilator and ICU bed capacity under pressure. Today, about half of hospitals using AI apply it to administrative areas like staffing, logistics, and inventory to cut costs and streamline workflows.
Diagnosis and Early Disease Detection
Predictive analytics is helping detect diseases earlier and more accurately. In fields like oncology and neurology, AI models trained on large datasets can flag risks for conditions like diabetes or cancer years before traditional methods.
In radiology, algorithms analyze imaging patterns to predict tumor aggressiveness or the chances of recurrence. AI also shows promise in rare disease diagnosis by spotting patterns in symptoms, genetics, and clinical data that humans might otherwise miss.
Public health agencies use predictive models to track outbreaks, like forecasting flu surges weeks ahead based on emergency room data and online trends. While some applications are still evolving, predictive analytics is already showing strong potential to support earlier diagnosis and better disease management.
Patient Engagement and Adherence
Some healthcare providers use predictive analytics to boost patient engagement by anticipating behaviors. Models can flag patients likely to miss appointments or skip medications, prompting clinics to take preventive interventions.
Analytics also help personalize outreach, predicting whether a patient responds better to texts, calls, or other forms of communication. Although less common than clinical risk predictions, these use cases are growing as healthcare shifts toward more patient-centered care.
Some hospitals and insurers now use AI models to flag patients who might need social services support, connecting them with community resources and extending care beyond the clinic walls.
What You Need to Implement
Implementing AI solutions for hospitals involves several critical steps:
- Data Quality and Integration: Consolidate EHRs, imaging, lab results, claims data, and patient-reported outcomes.
- Tools and Infrastructure: Use cloud platforms and advanced analytics tools, ensuring compliance with healthcare data standards.
- Workflow Integration: Embed predictive outputs directly into clinical systems like EHRs to assist real-time decision-making.
- Governance and Compliance: Maintain HIPAA-compliant infrastructure, encrypted data transfers, and access control protocols.
- Continuous Model Monitoring: Regularly validate predictive models to ensure accuracy and fairness across diverse patient populations.
The growing use of predictive analytics is also driving transformations in telemedicine trends, enhancing remote patient monitoring and preventive care strategies.

Challenges in Implementing Predictive AI Modeling in Healthcare
Despite the benefits, predictive analytics faces several challenges.Addressing these challenges is essential for expanding AI capabilities in areas like assistive technology in healthcare, which aim to enhance patient care and accessibility:
Data Quality and Silos
Predictive analytics needs clean, connected data, but healthcare data often isn’t. Records can be incomplete, coded differently, or scattered across disconnected systems. Models trained on flawed data give flawed results. Fixing this often means major upfront work — standardizing data, integrating systems, and sometimes tapping into external sources like regional health exchanges. Without it, reliable predictions stay out of reach.
Privacy and Security Concerns
Handling massive amounts of patient data brings serious privacy risks. Healthcare providers must follow HIPAA and other rules to keep information secure. Even with strong safeguards, public concern about “big data” use remains. Regulators are tightening oversight, too, requiring transparency about how AI models work and are validated. Meeting privacy obligations takes constant attention, investment, and staying ahead of evolving rules.
Algorithm Bias and Equity Issues
AI models can pick up biases hidden in historical healthcare data, like underestimating risk in groups who historically received less care. Left unchecked, this can widen health disparities. Studies show many hospitals don’t yet audit their predictive models for fairness. Fixing this takes deliberate work: building diverse datasets, testing models across patient groups, and reviewing outcomes with multidisciplinary teams. It’s a growing priority, but still a major challenge.
Lack of Explainability (Black Box Models)
Many powerful AI models can’t easily show why they made a prediction, making them harder for clinicians to trust. Simpler models are more transparent but sometimes less accurate. Ongoing research aims to make complex predictions easier to explain. In the meantime, healthcare teams need to either use more interpretable models or design clear ways to show which factors drive risk scores, helping clinicians feel confident using AI insights in real care decisions.
Integration and Workflow Challenges
Getting predictive analytics into daily hospital operations isn’t easy. Tech systems need to “communicate” (EHRs, scheduling tools, triage workflows), and alerts must reach the right people at the right time. Poor integration leads to low usage. Staff also need training and trust-building: AI is a support tool, not a replacement. Plus, alert fatigue is real — too many warnings, and people stop paying attention. Successful rollouts usually happen gradually, with careful workflow design and continuous fine-tuning.
Resource Constraints and Cost
Predictive analytics is an investment. Larger hospitals often have the budgets and staff to build strong programs; smaller ones might struggle. Cloud solutions and off-the-shelf AI tools help, but costs for licenses, training, and specialized staff still add up. Showing clear ROI (fewer readmissions, better throughput) can help justify the spend. Partnerships and data-sharing networks are emerging to help smaller organizations access predictive tools more affordably, but resource gaps remain a real barrier.
Despite the challenges, predictive analytics continues to gain ground in healthcare. Progress isn’t always smooth, but by improving data quality, strengthening privacy protections, addressing bias, and focusing on real-world integration, healthcare organizations can move closer to realizing its full value.
Unlock the power of AI predictive analytics with Beetroot
AI predictive analytics is helping healthcare move from reactive treatments to proactive, data-driven care. Hospitals and clinics are using it to predict patient needs, manage resources more efficiently, and intervene earlier, improving outcomes for both patients and providers. But unlocking this potential takes more than just technology. It means working with clean, reliable data, building models that are fair and tested, and making sure AI insights fit naturally into the way healthcare teams already work.
At Beetroot, we focus on creating healthcare solutions that solve real problems and make a real difference. Our teams combine strong technical skills with an understanding of what healthcare organizations need on the ground. We help hospitals, clinics, and HealthTech companies use predictive analytics in ways that are practical, responsible, and built to last. If you’re looking to improve patient care, run operations more smoothly, or bring new HealthTech ideas to life, we’re ready to help.
FAQs
How is AI predictive analytics used to improve hospital operations?
Hospitals use AI predictive analytics to forecast patient volume, optimize staffing, manage beds, and predict supply needs. Models learn from historical trends and real-time data to help hospitals schedule the right number of nurses and doctors, avoid overcrowding, and reduce wait times. Predictive tools also support supply chain management by forecasting inventory needs and help maintenance teams prevent equipment downtime. Overall, AI makes operations smoother, cuts costs, and improves patient experiences.
What’s the difference between AI predictive analytics and traditional clinical decision-making?
Traditional decision-making relies on clinician expertise and reacts to symptoms as they appear. AI predictive analytics looks ahead, analyzing large datasets to flag risks before problems surface. For example, it might predict that a stable-looking patient is at risk of deteriorating within 24 hours. While clinicians draw from experience, AI models learn from hundreds of thousands of cases, spotting patterns that humans might miss. Predictive analytics doesn’t replace clinical judgment — it adds early, data-driven warnings to help shift care from reacting to preventing.
Can small or mid-sized clinics use AI predictive analytics?
Yes. Many small and mid-sized clinics now use AI through cloud-based tools or built-in features in EHR systems. These services don’t require large IT teams or big budgets. Clinics can start small, like predicting missed appointments or identifying high-risk chronic disease patients, and expand over time. Privacy compliance remains essential, but vendors typically handle much of the technical side. By picking user-friendly, validated tools, even small practices can use predictive insights to strengthen preventive care.
What data is needed to implement AI predictive analytics in healthcare?
The foundation is EHR data — diagnoses, procedures, medications, vitals, lab results, and visit histories. Imaging data, lab and pathology results, and billing data can add more predictive power. Some models also use social determinants of health like housing or income levels to refine risk predictions. Wearable device data (like heart rate trends) and patient-reported outcomes are increasingly important too. For operational predictions, hospitals tap into scheduling records and equipment logs. Whatever the sources, high-quality, complete data is key.
Is AI predictive analytics compliant with HIPAA and healthcare privacy laws?
Yes, if implemented correctly. Predictive analytics must follow HIPAA rules around protecting patient data — using secure, encrypted platforms, limiting access to authorized staff, and monitoring how data is used. Many modern cloud services are HIPAA-compliant by design. Some projects de-identify data to reduce privacy risks, but clinical applications usually keep data tied to patients, making strong security even more important. Compliance teams should stay involved to ensure rules are met, but predictive analytics itself fits within the existing legal frameworks when handled properly.
Subscribe to blog updates
Get the best new articles in your inbox. Get the lastest content first.
Recent articles from our magazine
Contact Us
Find out how we can help extend your tech team for sustainable growth.